Mark Davies / May 2025
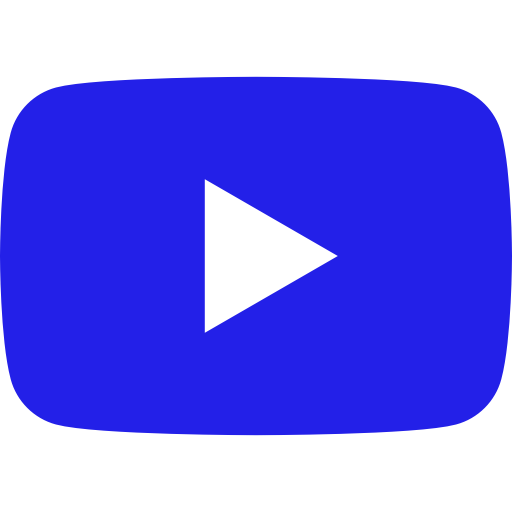
English-Corpora.org will soon offer
something entirely new: the ability to combine the depth and reliability
of corpus data with the analytic power of Large Language Models (LLMs)
like GPT, Gemini, Claude, Perplexity, Grok, Llama, and DeepSeek.
With just one click, the corpus will send
collocates, frequency patterns, phrase lists, or concordance lines to an LLM — which will instantly group, explain, and interpret the data. These
AI-powered insights appear directly in the interface, alongside the
original corpus results.
The result? Faster understanding of
patterns, clearer semantic groupings, and deeper insight into how
language works — for language learners and researchers alike. The corpus
data remains front and center, but now with the option of an intelligent
assistant working behind the scenes to guide your analysis.
Corpora first. AI second. Insights
faster. |
Background: In March 2025 I released seven detailed studies (with a total of 89
pages of discussion and examples) discussing how well the
predictions of two LLMs -- GPT-4o (from OpenAI) and Gemini 1.5 Pro
(from Google) -- match the actual data from large, well-known,
publicly-accessible corpora (like those from English-Corpora.org). The seven
detailed studies are found here:
word frequency,
phrase frequency,
collocates,
comparing words (via collocates),
genre-based variation,
historical variation, and dialectal
variation. You can also see a summary of
these findings, as well as a
video on YouTube.
Soon after I finished this comparison,
however, I thought -- maybe it's not a question of "either/or".
Maybe it's a issue of "and/with". Why not take the strengths of AI /
LLMs, and integrate them right into the corpus interface? As the
comparison between corpora and AI/LLMs
indicate, what LLM are really good at is classifying and
explaining data. And that's why this insight is being directly
integrated into
English-Corpora.org.
The following are some of the ways that the AI/LLM insights
are being integrated with corpus data. Pay close attention to the
categorization and
especially the analysis from the LLMs. None of the analyses that you see on any
of these pages are human-generated; they all come from the LLMs. I think you'll
agree that this insight from LLMs will completely transform the way that people
interact with the corpora, especially for non-native speakers and language
learners.
(Of course, while the AI analyses offer powerful
insights, users should remember that they represent intelligent suggestions
based on patterns in the data, and they are not 100% accurate linguistic
conclusions. If you demand absolute perfection and accuracy, then LLMs might not be for you.)
Note that I already have this functionality
working in in the corpora (that's how I got the data shown below). I will then release it for "beta testing" in June
(and you can volunteer to help with that),
and then for public release in August 2025.
Task / function |
Examples / discussion
(note: there are more examples in the video) |
Video |
PDF |
Introduction: Integrating LLM insights and corpus data |
The mechanics of integrating LLM insights into corpus
data |
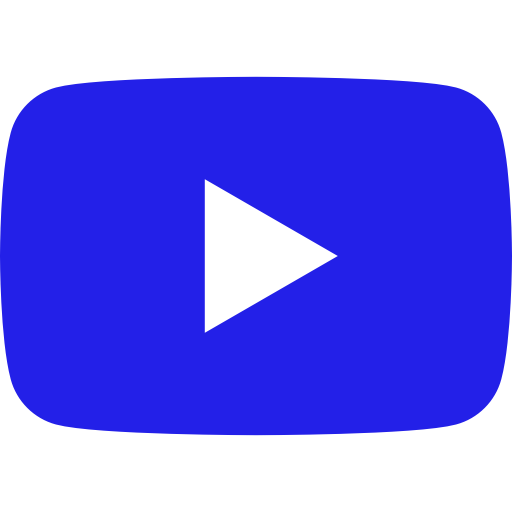 |
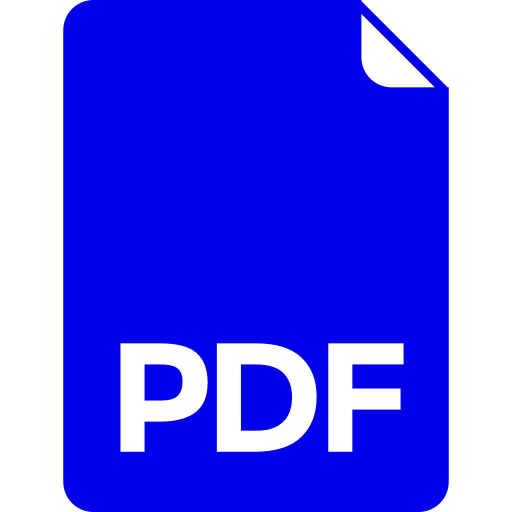 |
Classifying and categorizing collocates and related
phrases |
Collocates of cap, collocates of bow
(COCA, iWeb), soft NOUN |
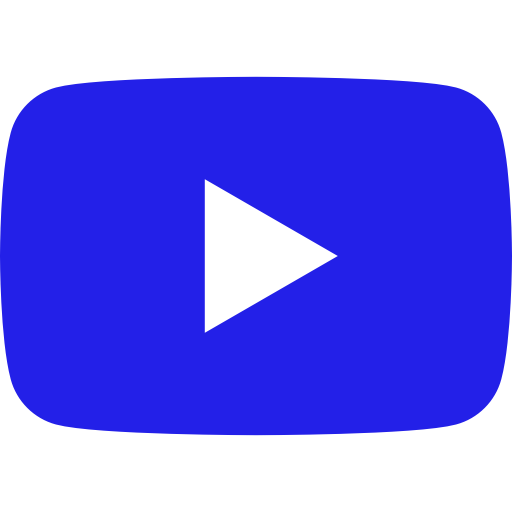 |
|
Comparing two words (via collocates) |
Quandary vs predicament, provoke vs
incite, fleeting vs ephemeral |
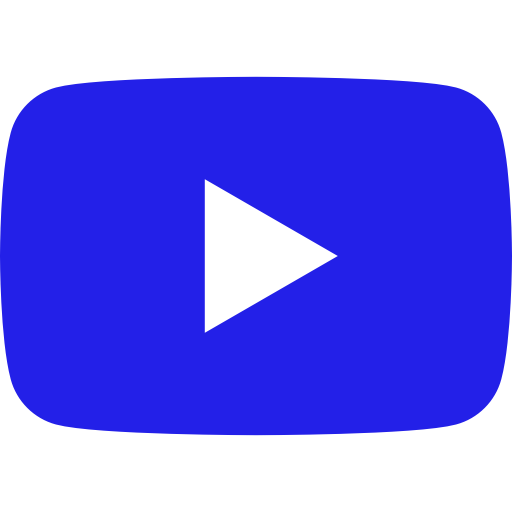 |
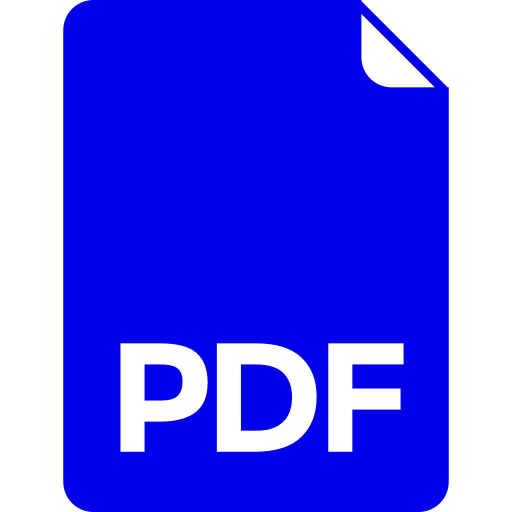 |
Comparing two genres, time periods, and dialects
(lists) |
chain + NOUN (fic / acad), ADJ women
(1800s / now), ADJ scheme (US / UK) |
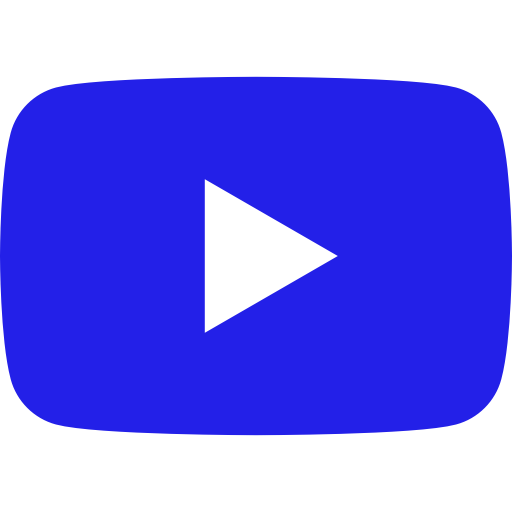 |
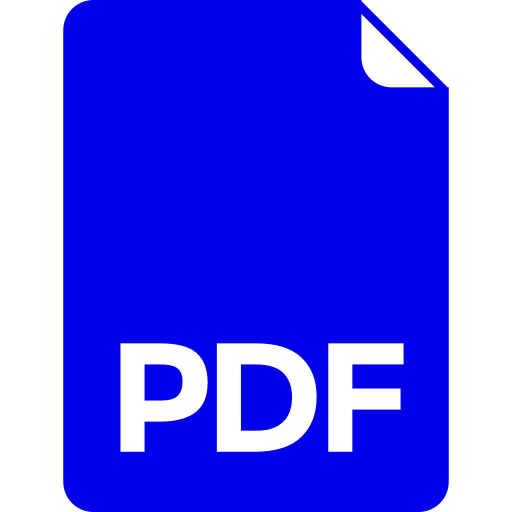 |
Comparing genres, time periods, and dialects (all
sections) |
soft NOUN (genres), ADJ food (historical), *ism
(dialects) |
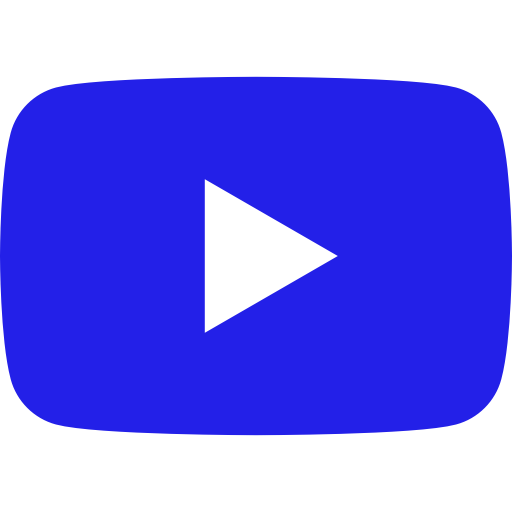 |
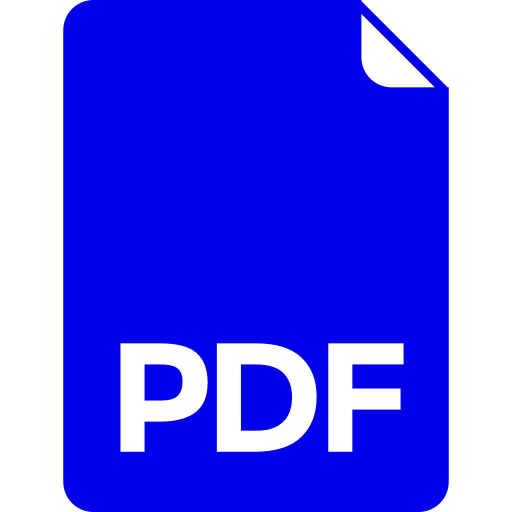 |
Comparing genres, time periods, and dialects (charts) |
"like construction" (genres), need NEG (historical),
soft day (dialects) |
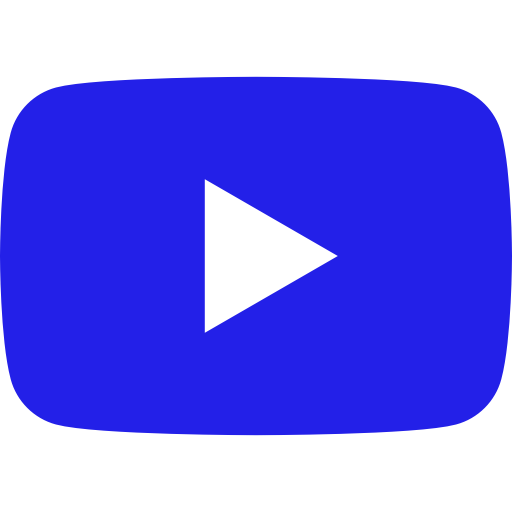 |
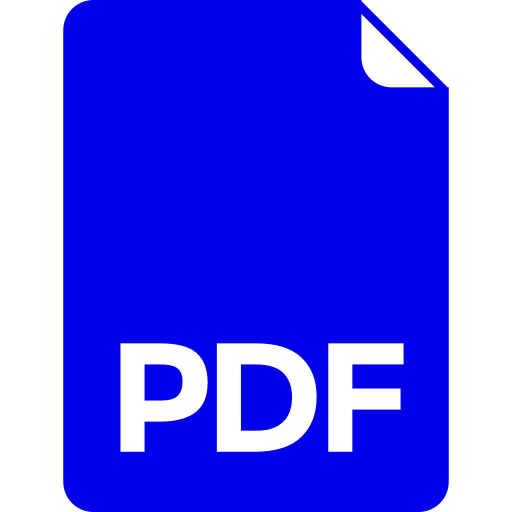 |
Analyzing KWIC/concordance lines |
fathom, naked eye (collocations, prosody,
syntactic, pragmatic, domains) |
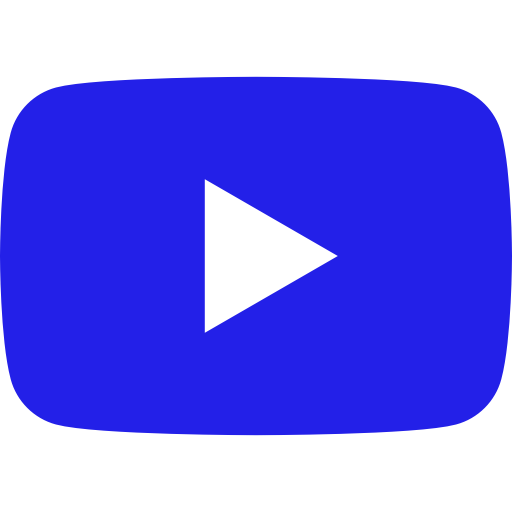 |
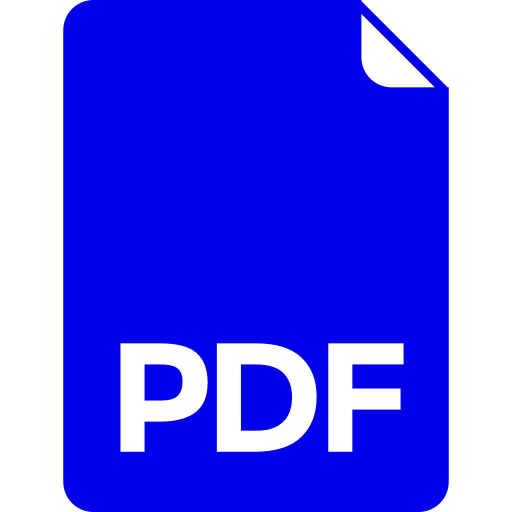 |
Generating words and phrases for topics and concepts |
astronomy, COVID-19, female jobs in 1800s, "I'm tired",
Sp manguera, Ger es tut mir leid |
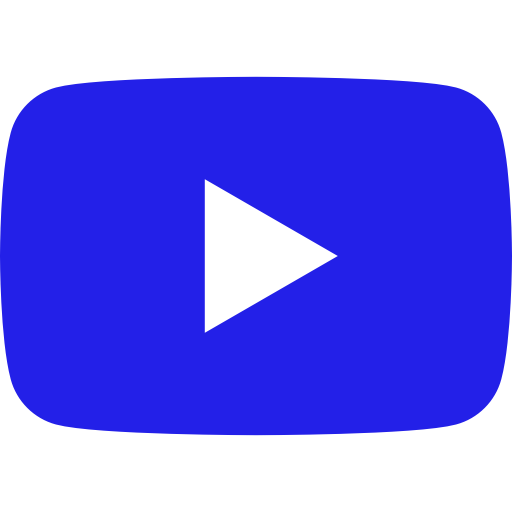 |
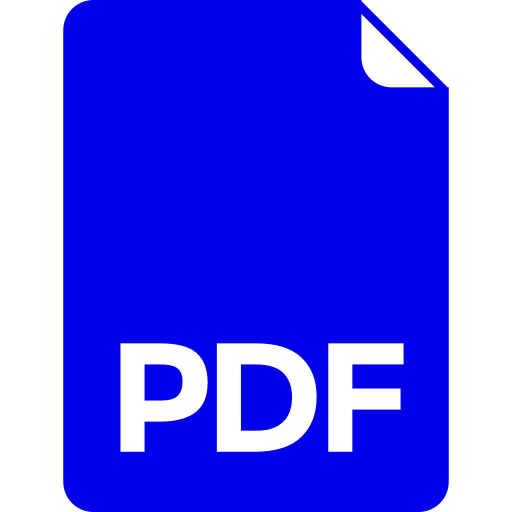 |
Conclusion |
|
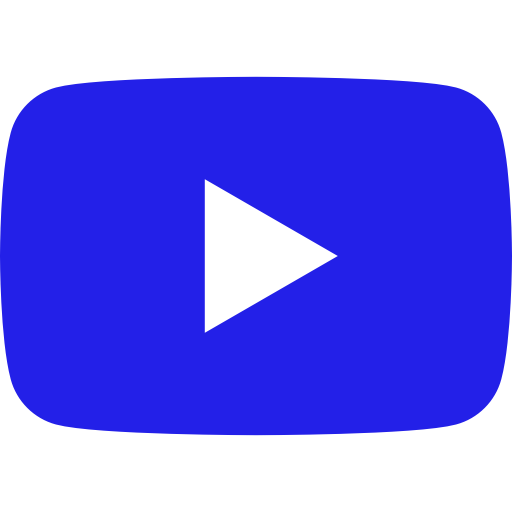 |
|
|